Geological knowledge discovery using machine augmented intelligence
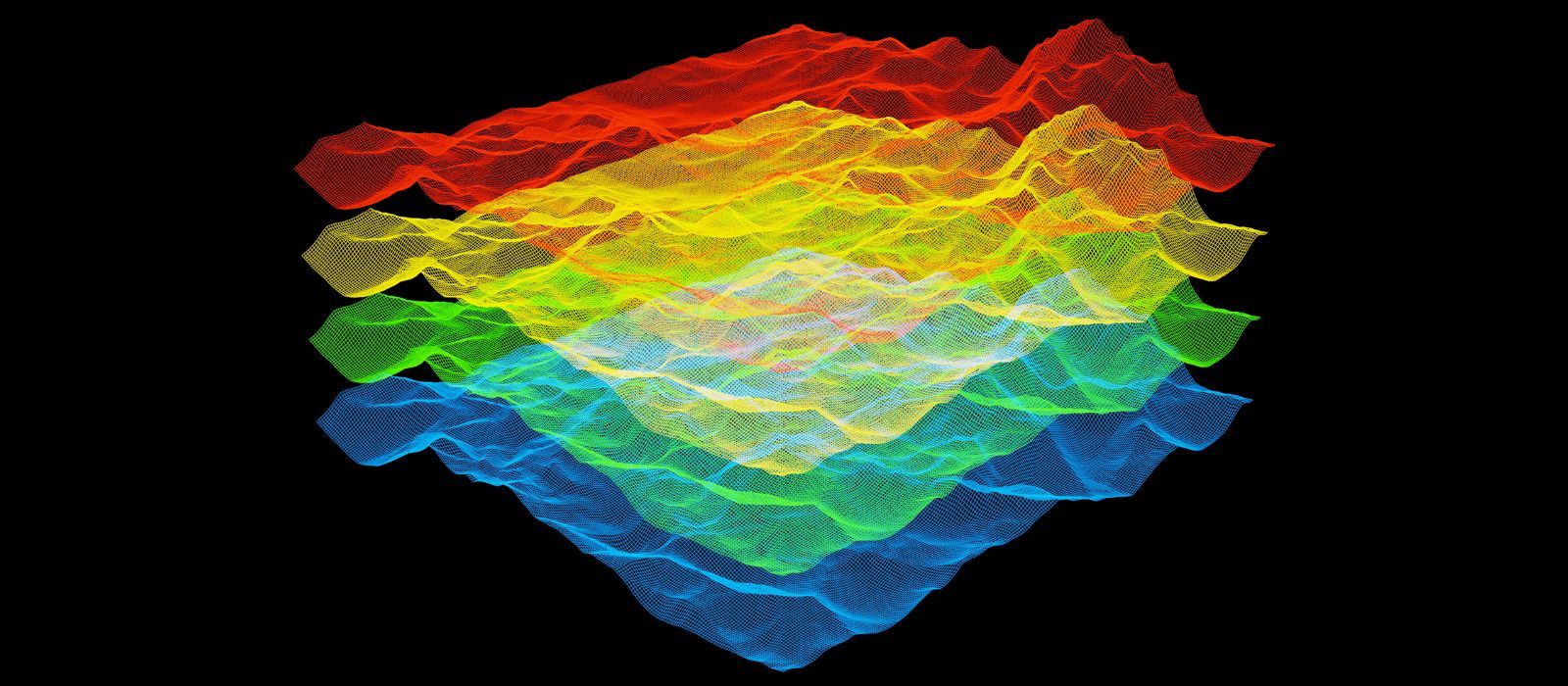
Exploring how the power of automation and artificial intelligence (AI) can help geoscientists make informed geological interpretations
Geological interpretation is a complex task, where an interpreter’s bias has a significant impact. As a result, interpretation outcomes are variable and uncertain, but these outcomes form the basis of critical decisions at different stages of the mining value chain including mineral exploration, resource modelling, and mining.
With the increasing adoption of artificial intelligence (AI) and machine learning in our daily lives, such as for online searches, online shopping, and virtual assistant AI, the geoscience domain has also been active in the uptake of machine learning and AI to assist in interpreting geology from data.
You might also like
The Centre for Data-driven Geoscience (CDG) at the University of Western Australia has been working closely with the minerals industry to develop data science applications over the past 15 years. These applications use automated image analysis, machine learning and AI to extract geological information/patterns and knowledge from diverse types of data, including regional scale geophysics images, mine scale drill hole data and geological text. While the automated solutions give fast, consistent and reproducible outcomes, disseminating these technologies to help end-users make decisions in actual industry practice is challenging. The main challenges include addressing the decision maker’s trust in data science solutions; and the usability of the machine augmentation in industry practice.
Building trust in automated solutions
To help build trust in the solutions presented to end users, it is important to consider the transparency of the algorithmic process and the impact of parameters used in the algorithm; this will help an end user make a more informed decision about outputs from the algorithmic process. It is also important that the solution communicates as clearly as possible with the user the uncertainty or variability of the outcomes, such as the impact of the input features to the output and its certainty.
Improving useability
Improving the useability of automated solutions may involve addressing the potential pitfalls in generating ‘false positives’, which require a time-consuming QA/QC process to identify and remove. It is also important that automated solutions are properly integrated with existing data interpretation workflows and other software platforms that are currently being used. Finally, it is important to consider the transferability of the methods or machine learning models that have been tested successfully in one geological environment to a new environment.
The CDG team’s research addresses these trust and usability challenges by employing a human-driven and machine-assisted approach. The three case studies presented below help explain successful machine augmentation for geoscience end users.
Automated solutions in action
The first case study is the televiewer image analysis system, which incorporates automated feature detection techniques that automatically pick geological structures into an extensive interactive workflow to ensure the uptake of the automated outputs. This involved allowing the user to control the algorithm’s process and outputs interactively, including filtering and modification of outputs, to categorise different types of structures. This also helped meet the requirements of different levels of detail required for different end user groups, for example structural geologists and geotechnical engineers.
The second case study is machine-assisted stratigraphy interpretation using multi-modal drillhole data, specifically wireline gamma, geological logging and geochemical assays. Deep learning-based classifiers are developed for each data modality, and their outputs are used in an interactive visual analytical platform to find the stratigraphic sequence within each drillhole. This platform allows the analysis and integration of machine learning outputs with auxiliary datasets routinely used for interpreters, along with the geologically constrained optimisation process to trace a likely stratigraphy sequence along the drillhole while visualising them in 3D context.
The third case study is ongoing AI research that extracts geological insights from documents using machine reading of text. It applies advanced text mining methods, based on natural language processing and deep learning, to construct a graph-based knowledge base (called a knowledge graph) to store and access geological information and relationships. The method was successfully applied to reports from different mineral deposits, and demonstrated the realisation of machine augmentation in validating or bring new insights for mineral exploration.
Conclusion
The above three case studies show how machine augmentation is applied to alleviate bottlenecks in industry where a large amount of data needs to be analysed with limited resources, and to improve consistency and robustness of data interpretation. However, they also show that machine augmentation is only effective if it can be integrated into a workflow with a specific aim to improve the trust and usability of machine solutions.
This article is based on a recent Geoscience Society technical talk.
Related content
To get more value from your operation through data analytics, machine learning, simulation and optimisation, explore the Practical Data Analytics and Machine Learning short course.