International Mining Geology Conference – Abstract Showcase
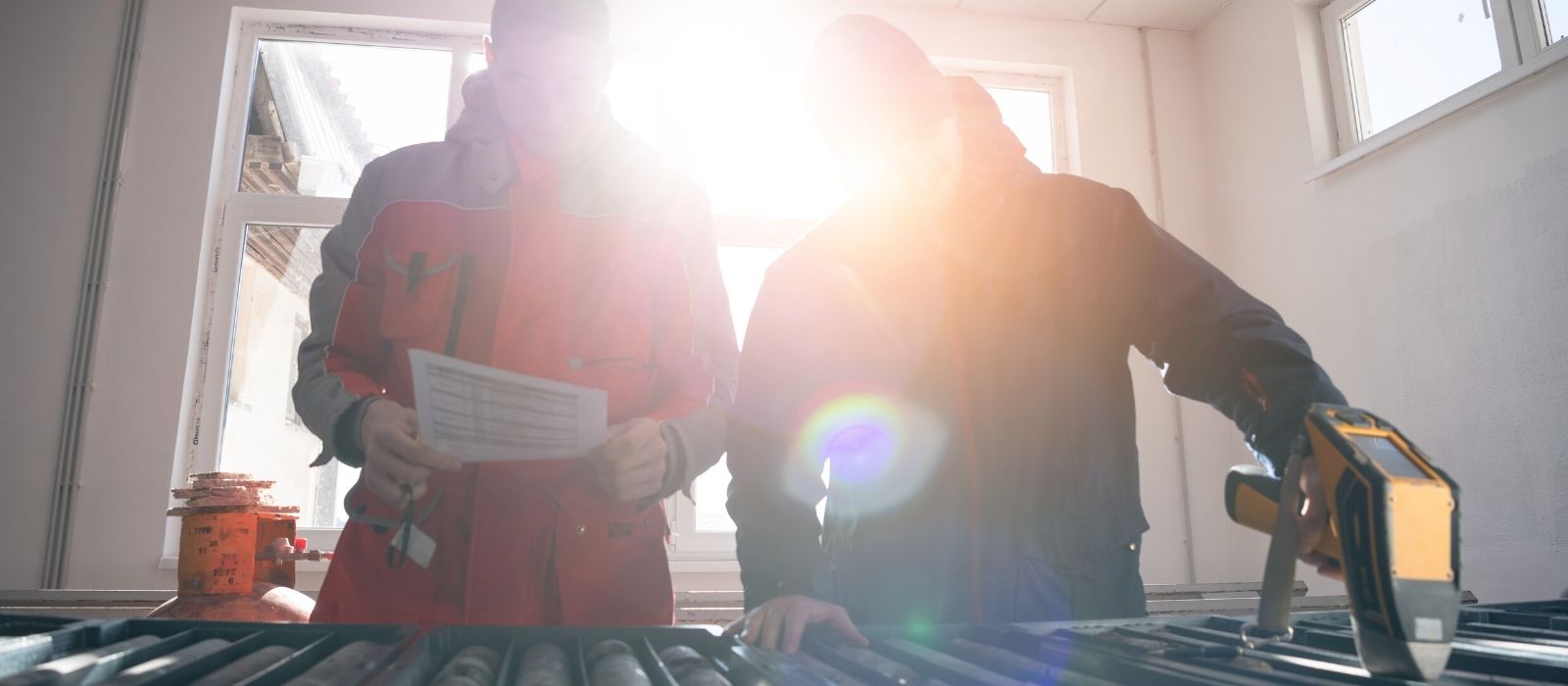
AusIMM’s International Mining Geology Conference 2022 (22-23 March, Brisbane and online) will bring together leading industry experts to share their knowledge on new techniques and emerging technologies.
This article showcases abstracts of papers to be presented at the upcoming conference. The abstracts chosen cover reconciliation, fundamentals of resource estimation, grade control and software and technology. They represent the latest thinking in the field of mining geology, written by experts with a deep understanding of their field.
These are just a small sample of the content that will be on offer at the conference, and we encourage anybody interested in these papers to view the full list of abstracts on the conference website to discover more insights.
You can also register to attend a free pre-conference webinar on 9 March by Rayleen Hargreaves on Mine Reconciliation Standardisation - R Factor Series.
Camp scale structural influence on the Ernest Henry orebody below current mining levels
E Philippa, Geologist, Ernest Henry Mine and B Miller MAusIMM, Principal Geologist, Gnomic
Abstract
The Ernest Henry Iron Oxide Copper Gold deposit is hosted in a large dilation between two moderately dipping shear zones found in the foot wall and hanging wall of the deposit. These shears are controlled by a large diorite intrusion to the North-East and South-West of the deposit, formed ~1660ma.
A shear zone dubbed the ‘Interlens’ uncovered through underground workings truncates the main ore body into two lenses, and is hypothesized to have formed in conjunction with the Hanging Wall Shear Zone and Foot Wall Shear Zone. All three shears dip moderately to the SSE (50-140) from open cut exposures through to the current mining horizon approximately 800m below the surface.
Routine geological work and a capital drilling campaign has identified a significant flexure in these sub parallel ore bounding shears with increasing depth. A shift in shear orientation in the Interlens over approximately 100m vertically from ~50° to the SSE to a steep, easterly trend (~85° to the East) is observed in both drill core and exposures in the underground workings. It is hypothesized the diorite intrusive to the South-West of the deposit controls the shear zone geometries at depth. The geometry of the Interlens mirrors the surface expression of the Proterozoic package of the orebounding shears to the SW of the deposit, suggesting the surface geometries of the shear zones are consistent with depth.
Additional drilling to explore the validity of this hypothesis is planned for the near future to better understand the structural controls on the Ernest Henry mineralization with increasing depth.
Machine Learning in Resource Geology: Why Data Quality is Critical
F A Pym, M P Murphy, K E Crook and P M Hetherington
Abstract
The Nova-Bollinger Deposit is magmatic sulphide nickel-copper-cobalt deposit hosted in the Albany Fraser Orogen, approximately 160km east-northeast of the town of Norseman in Western Australia. Since mining commenced in 2016, the Nova Operation (Nova) has mined and processed 5.63Mt of ore grading 2.04% Ni, 0.86% Cu and 0.07% Co to 31 December 2020.
The 2020 update of Nova-Bollinger JORC Code Mineral Resource estimate (MRE) was completed by using well known implicit modelling and estimation software. The MRE is modelled on data from ~386km of predominantly diamond core drilling, with drilling having nominal grid spacing of 12.5 by 12.5m throughout the mineral resource volume. Twenty-two domains were modelled from the data using implicit wireframing tools that incorporated strings generated from underground mapping where available. During 2021, a new machine learning (ML) application was trialled to compare the ML results to the current workflow Nova for modelling estimation zones. The ML application was provided with the same drill hole data used for the current MRE to determine if a similar result to the implicit modelling could be achieved, and if a practical estimate could be prepared. ML requires a different approach to overcoming the usual challenges caused by inconsistencies in drill hole data. Compared to the industry standard approaches of wireframing or implicit modelling, where the modeller usually makes many subjective choices to produce the estimation zone model, ML offers a more objective ‘hands-off’ process to determining the connectivity of estimation zones defined in drill holes. This paper details the results of the ML modelling trial at Nova and the lessons learnt along with ideas for future work.
Creating optimized drill programs that add real value, and integrate with mine planning
N Anderson MAusIMM
Abstract
Using new technology to create optimised drill program designs, it is possible to quantify the value of a drill program. The computer designed programs are optimised to uplift the most amount of resource possible into the specified resource classification, while honouring geological, spatial, equipment and budgetary constraints.
When designing ‘by hand’, balancing all of these factors is a time-consuming effort with no real way to determine metrics on ‘how good’ a program will be. The optimisation considers many factors allowing geologists to apply critical thinking and domain knowledge to constrain the optimisation, letting the computer do the heavy lifting of determining hole placement.
Once programs have been created, they can be integrated into a production schedule. This is a critical step in determining the correct sequencing and balancing of resource availability and seeing how a program will interoperate with other tasks in the mine. For example, if drill drives are a requirement for part of the program, will they be developed in a timeframe that is useful for the goals of the program? Do we risk drilling infill holes too late to add value? Do we put production at risk by not classifying parts of the model ahead of time, thus adding to uncertainty?
Using software to assist with optimising and scheduling of drilling is a useful technique to save the geologists from the mundanity of manually laying our drill programs and puts metrics around the effectiveness of a program and has the potential to add quantifiable value to a program.
Several examples will be presented that demonstrate the effective use of drillhole optimisation, comparing manual designs with optimised designs, as well as the examples that show the effective use of scheduling to manage limited availability of drill locations.
Empirical Geostatistics # 1: Kriging Slope of Regression – Sensitivities and Impacts on Estimation, Classification and Final Selection
D J Kentwell, SRK Consulting
Abstract
With the advances in software speed and capability, many of us now are running multiple scenarios on entire models rather than just testing small areas or a few blocks. In the author’s experience, both the geological and geostatistical academic theory and our rules of thumb often prove un-useable or incorrect in the real world we work in. The only way to truly validate and tune our models is to complete the entire model and ‘see if it works’. If it lacks some property we were expecting, then we need to find out why and/or run different scenarios to see what changes. This is not always possible with tight project timelines, but we find ourselves doing more and more of this sort of thing, and in so doing, we understand that every deposit is different and requires its own ‘rules’ to get valid and useful results. For the purposes of this paper, this process is termed Empirical Geostatistics.
Take Clayton Deutsch’s ‘all realisations all of the time’ concept a step back. Before you even think of simulating, test the alternate realisations generated by alternate parameters such as different domaining, varying search neighbourhood parameters, etc. (then, if you want, increase the number of simulations required….as you simulate each alternative…..)
Topic #1: Kriging Slope of Regression
Never has a statistic been more misunderstood, misused and abused than the poor old kriging slope of regression.
- Maximise me! (No, you’re an oxymoron)
- Classify me – I don’t care how!
- Block size with me – bigger is always better (really?)
- Drill space with me (but please don’t de-cluster me)
- Don’t top cut me, threshold me (Actually it doesn’t matter – or does it?)
This paper takes a fresh look at the humble kriging slope of regression and its close friend, kriging efficiency (KE); and examines, and shows examples of, its misuse and misunderstanding, while tying these back to potential impacts on classification, grade and tonnage estimates and final selection outcomes.
Applying supervised machine learning and multiscale analysis on drill core data to improve geological logging
F Huang, E J Hill and A Otto
Abstract
Geochemical data from drill core samples is used in mineral exploration to enable geologists to characterise subsurface geology. However, it is challenging to analyse these data sets rigorously and consistently. Manual data interpretation relies on the knowledge and experience of logging geologists. When multiple geologists are involved, it is possible to introduce inconsistencies in logged lithology type and scale of logging (“splitters” versus “lumpers”). Machine learning (ML) is a powerful tool for analysing high dimensional data (i.e. many variables) and large data sets (i.e. many samples). However, ML methods applied to drill hole samples do not incorporate spatial information and this can result in high misclassification rates and small-scale “noisy” results. We demonstrate the use of multiscale spatial analysis (wavelet tessellation) to incorporate spatial information into automated logging of drill core to help reduce misclassification and filter out unwanted small-scale variation in the data.
An experiment combined the use of wavelet tessellation and supervised ML to predict the rock types from two geochemistry datasets: (1) Valhalla uranium deposit in the Mount Isa Inlier, Queensland, and (2) Sunrise Dam gold mine, Western Australia. First, geochemical knowledge and statistical analysis was used to select appropriate geochemical elements for litho-geochemical classification. Second, the elements were processed using multiscale spatial analysis. Third, several ML algorithms were tested, including logistic regression, support vector machines and several tree models. We found that all ML algorithms behave worse in predicting rare rock samples than common ones. Therefore, we developed a statistical model to synthesize samples of rare rock types to overcome this issue. Results show that Random Forest has the highest accuracy of prediction. We conclude that the combination of multiscale spatial analysis (to define rock units) and Random Forests (to classify rock units) is a good method for rapid and accurate analysis of drill hole geochemistry.
Mine Reconciliation as a Driver of Improvement: Best Practice in Reporting and Analysis
R R Hargreaves, T Elkington and A Cook, Snowden
Abstract
Mine reconciliation is now recognised as a vital standard periodic process at most mine sites, with most systematically preparing and delivering monthly reconciliation reports. But every site tends to report and analyse their reconciliations slightly differently. The authors have been exposed to many versions of monthly reconciliation reports, ranging from 2 pages to 80 pages per month. Producing an 80-page reconciliation report is time-consuming for your valuable personnel, particularly when it is derived from numerous Excel spreadsheets.
But what happens next? Should not there be learnings and follow ups from the results? Why waste the effort in preparing the data for reporting when it can be used to better inform forecasts and deliver improved reconciliation going forward?
Using best practice reconciliation principles, this paper will provide guidance for standardised reporting analysis and more importantly, explain how we can go beyond standard reconciliation reporting to identify sources for improvement.
Through a case study, the authors explore best practice in reconciliation report and analysis for (a) reconciliation factors, and (b) compliance to plan. The authors will demonstrate that by using a rolling factor across time, improvements to the individual data sources can be easily identified. Using the methods and learnings from geostatistics, the authors will demonstrate that different types of plots can be used to provide different types of outlier detection and prompt follow-up. With a systemised focus on improvement, reporting and analysis can be streamlined to enable more time to be spent on improvement and less on manual data manipulation and report generation.
More information
AusIMM’s Mining Geology Conference is taking place in Brisbane and online from 22-23 March, 2022. Visit the website to find out more and register today.