Measuring rehabilitation success using remote sensing
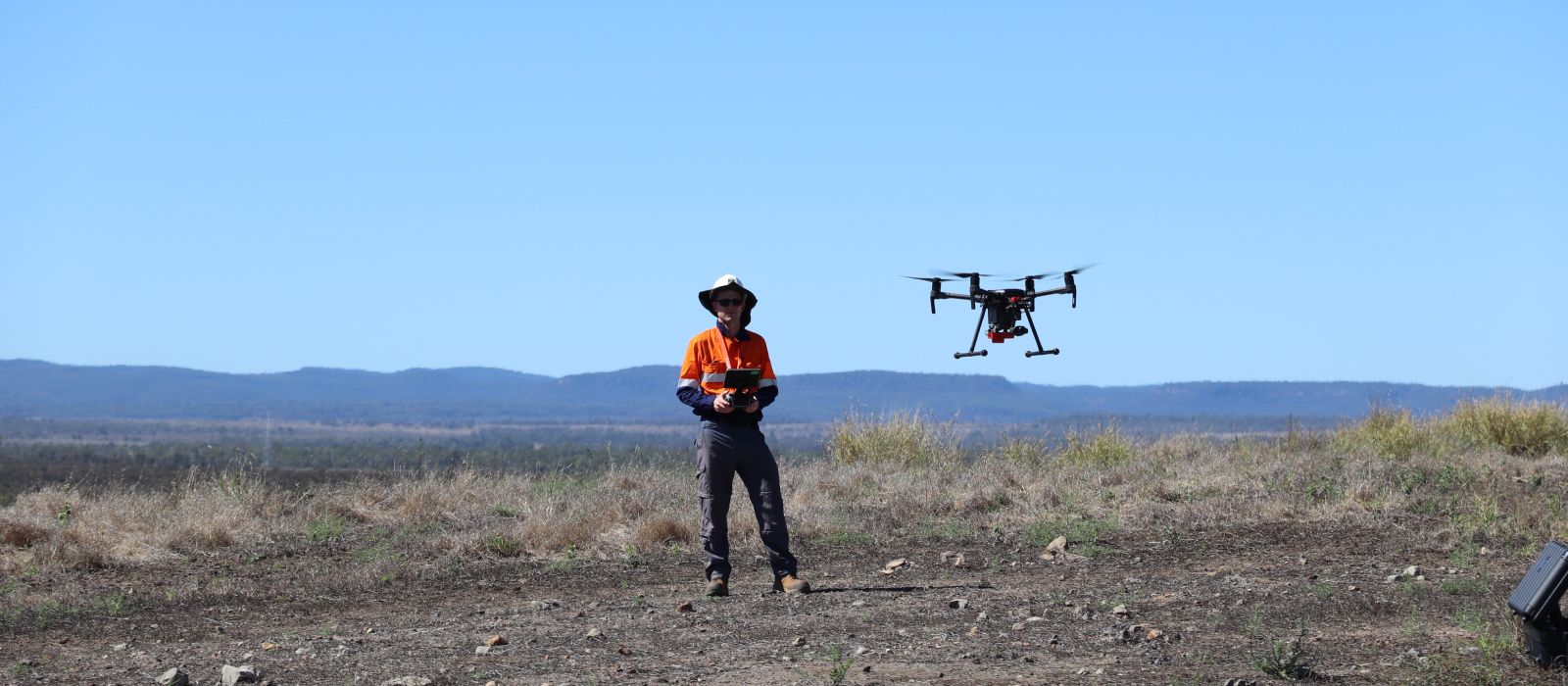
Remote sensing is becoming the tool of choice for mine professionals to measure progressive rehabilitation, plan for future work, and demonstrate success for certification and mine closure.
In this article, we discuss remote sensing approaches to environmental monitoring, and consider options for assessing ecological attributes to quantify rehabilitation outcomes.
What is remote sensing?
Remote sensing is the measurement of target features of interest from a distance, most commonly using imagery and data captured from satellites, aircraft and drones. These crafts use either ‘passive’ sensors that capture reflected optical sunlight, or ‘active’ sensors that produce pulses of energy (eg laser, sonar or radar) to map and measure a range of target features such as vegetation vigour and landform stability.
What can remote sensing measure?
Over the last 50 years, the number of scientific studies applying remote sensing technology to rehabilitation assessments have been increasing. The science has been dominated by coarse spatial resolution sensors such as Landsat (30 m pixel size), but in the last decade a significant shift has resulted in the use of more complex remote sensing platforms and sensors such as drones and high resolution earth observation satellites (McKenna et al, 2020) (Figure 1).
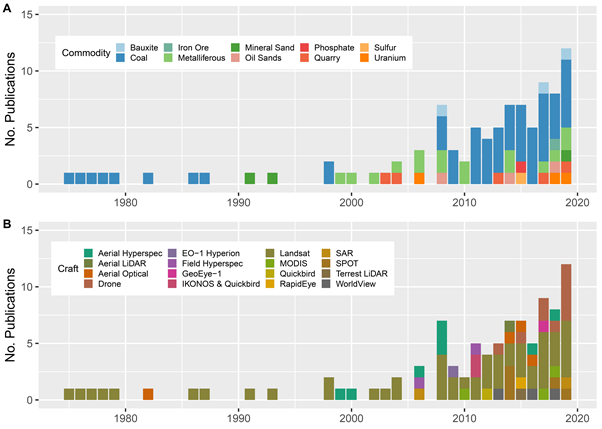
To determine the potential for remote sensing to monitor rehabilitation success, we used the ecological monitoring wheel published by the International Society for Ecological Restoration (SER) (Gann et al, 2019). Initially designed for comparing restored ecosystems with reference communities, we modified the tool to assess the suitability of remote sensing for monitoring each ecological attribute. We found, using a scale of 1 to 5, twelve of the total eighteen sub-attributes are highly suited to remote sensing; four have low to moderate suitability and two sub-attributes are not suited to remote sensing (Figure 2).
In the following section we provide examples of potential applications of remote sensing in mine-site rehabilitation for each of the ecological recovery wheel’s attributes, and discuss the most appropriate sensors for each metric.
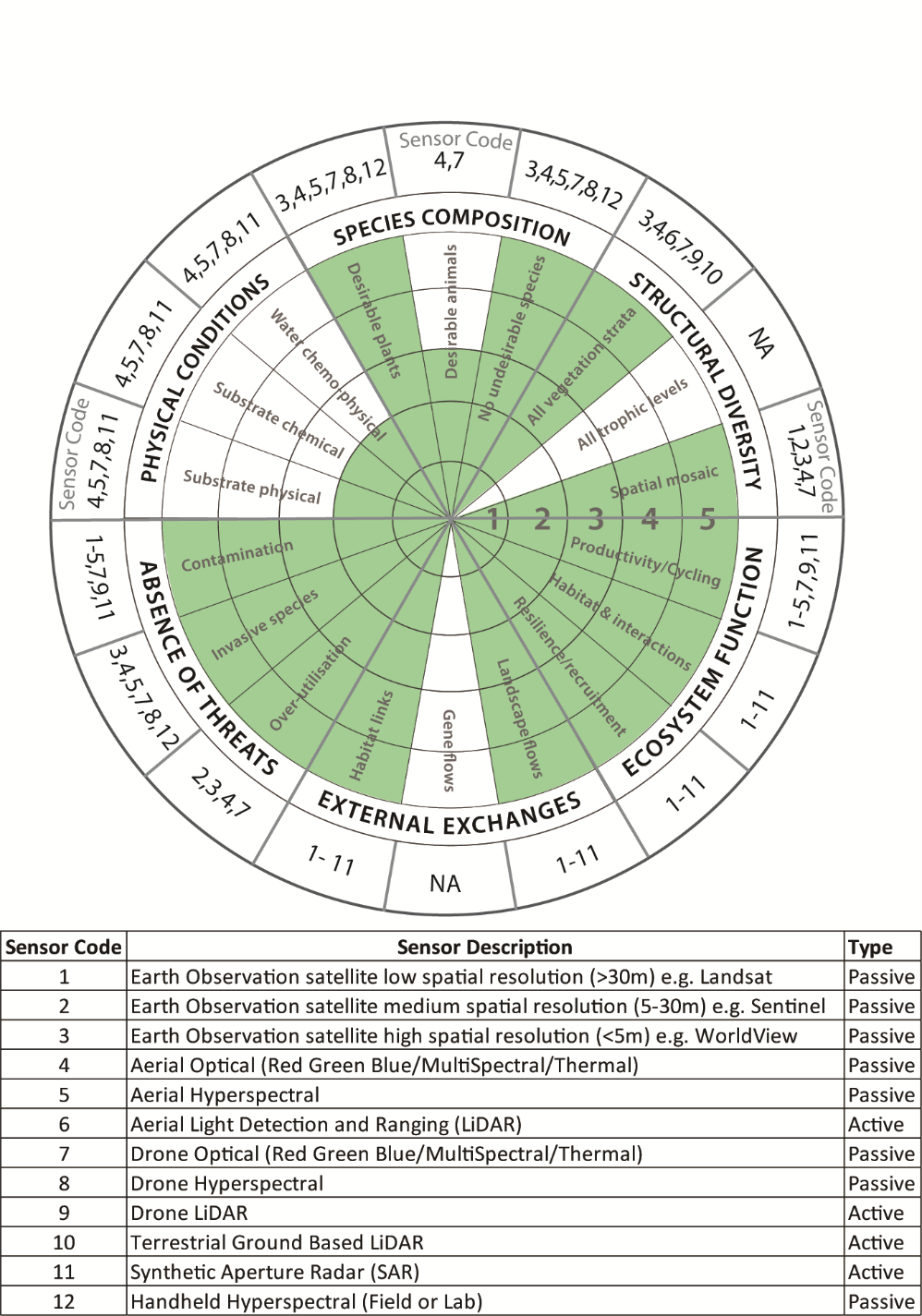
Species composition
Measuring the levels of desirable and non-desirable vegetation is possible using high-spatial resolution multispectral/hyperspectral imagery and Light Detection and Ranging (LiDAR), either in single images or as a time-series. Individual species can be detected and measured based on unique spectral signatures and biophysical features such as vertical height and shape (eg canopy arrangement).
For example, many weed species have unique colouring, sizes and shapes that can be detected and mapped (Figure 3). Desirable and non-desirable animals may also be detected successfully using high-resolution thermal imagery (eg kangaroos, koalas and feral pigs).
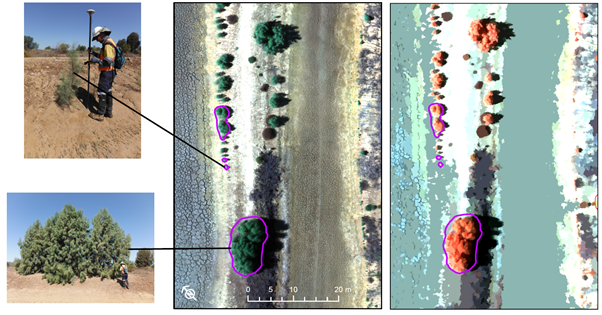
Structural diversity
Remote sensing is highly suited to quantifying vegetation strata and cover and is one of the most common approaches used in rehabilitation monitoring. Vegetation cover can be easily assessed using optical sensors at a range of spatial and temporal scales. Vegetation strata includes key species in the canopy, mid-storey and at the ground level, and point clouds derived from LiDAR sensors are best suited for this purpose.
Ground-based terrestrial LiDAR sensors are also capable of measuring biomass and vegetation structural features at high accuracies, but are labour intensive; while point clouds from optical drone imagery are capable of providing high-resolution information on terrain features including vegetation height.
Ecosystem function
Using a range of sensors for the assessment of ecosystem function is highly achievable using the sub-attributes productivity/cycling, habitat and interactions and resilience/recruitment. In particular, multispectral and hyperspectral imagery are particularly suited to quantifying vegetation growth and vigour, nutrient cycling, vegetation chemistry/moisture, species interactions and disturbance recovery.
Rehabilitation resilience and recovery from disturbance requires time-series imagery collected over a longer temporal scale. While Landsat is the sensor of choice for long (decadal) time-series (incorporating a free image library from the 1970s), relatively new high spatial (3 m) and temporal (daily capture) satellites such as PlanetScope are well suited to quantifying changes in ecosystem function over time. Productivity metrics such as plant biomass have also been measured using the increasingly popular sensor Synthetic Aperture Radar (SAR).
External exchanges
Landscape flows and habitat links are well-suited to mapping using a wide range of passive and active sensors. For example, rehabilitation may support ecological connections (eg wildlife corridors, offsets) between unmined vegetation, which could be mapped using earth observation low, moderate and high multispectral sensors.
Absence of threats
Invasive species, contamination and over-utilisation are all well-suited to measurements using multispectral or hyperspectral imagery. Identifying and managing weeds is becoming a common approach for remote sensing, particularly those species easily identified in imagery due to distinctive colour and shape such as Parkinsonia and Athel Pine (Figure 3).
Additionally, contamination via mineral accumulation in foliage and accumulation of uranium bearing dust from tailings dams on surrounding vegetation has been measured successfully using hyperspectral imagery (Weiersbye et al, 2006).
Physical conditions
Substrate assessments such as soil moisture, pH and salinity are possible with SAR, hyperspectral and thermal sensors; however, such assessments become increasingly difficult with the presence of high biomass vegetation and high heterogeneity in soil and spoils common in rehabilitated landscapes. Landform stability (slope, erosion) is commonly assessed using LiDAR and drone derived point cloud photogrammetry.
Scale of applications and importance of workflows
Many mine sites already have the capacity to conduct in-house remote sensing assessments and this is likely to become more common across the industry as new user-friendly online tools emerge. Aerial imagery and LiDAR is captured across most sites either annually or monthly, and the use of drones for mapping rehabilitation is increasing (Figure 4). At a minimum, site staff are able to use this imagery along with other free imagery resources such as Sentinel or Landsat to demonstrate time-series changes and rehabilitation development.
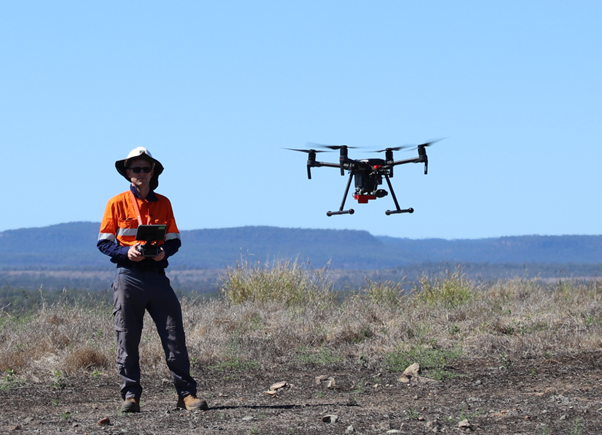
A number of commercial and non-commercial software applications are capable of automating workflows to quantify a range of ecological attributes listed in Figure 2. For example, once trained for a specific site, re-running an existing workflow to calculate indices indicative of vegetation vigour or woody stem density is relatively straightforward, making future reporting much less labour intensive. As a result, remote sensing workflows are now a powerful reporting tool to demonstrate to stakeholders the success of rehabilitation development and the achievement of closure criteria. Note that the choice of sensor to support a remote sensing monitoring workflow will be highly dependent on the physical and temporal scale required for the project and therefore the area of interest and features to map and measure.
What are some key points relevant for rehabilitated landscapes?
While there are common remote sensing applications for forest ecosystems (Lechner, Foody and Boyd, 2020), there are a number of factors specific to post-mine rehabilitated landscapes that are important to consider:
- Mine rehabilitation is a spectrally unique target. As a constructed landscape, rehabilitation is heterogeneous and often includes discrete patches of vegetation in different stages of development. Underlying soil characteristics and topography can vary significantly over short distances, particularly when compared to neighbouring unmined land. Additionally, rehabilitation managers often vary seed mixes, topsoil depth, and site preparation techniques, resulting in high levels of spectral heterogeneity within and between patches and across post-mining landscapes.
- Increases in vegetation cover measured in imagery does not equate to rehabilitation success. Many studies wrongly assume that an increase in vegetation index values (eg Normalised Difference Vegetation Index (NDVI)) or vegetation cover demonstrate measures of rehabilitation success. NDVI is the most common index used across the literature, but this index is highly variable according to seasons, daily rainfall and background soil effects.
- Ground points to calibrate and validate remote sensing outputs are still very important. While remote sensing may aim to replace ground based ecological monitoring, it is best practice to validate results and users should be wary of remote sensing products that do not quantify overall map accuracies and provide confidence levels for ecological metrics.
Conclusion
This article has discussed just some of the remote sensing technologies and approaches available for rehabilitation monitoring in the mining industry. With improvements in technology and ease-of-use, it is becoming more and more likely that remote sensing approaches will become a standard tool for resource professionals to successfully monitor post-mine land rehabilitation and help create better outcomes for the industry and communities.
Author information
References
Gann G D, McDonald T, Walder B, Aronson J, Nelson C R, Jonson J, Hallett J G, Eisenberg C, Guariguata M R, Liu J, Hua F, Echeverría C, Gonzales E, Shaw N, Decleer K, Dixon K W, 2019. ‘International principles and standards for the practice of ecological restoration. Second edition’, Restoration Ecology, 27(S1), pp. S1–S46. doi: 10.1111/rec.13035.
Lechner A M, Foody G M and Boyd D S, 2020. ‘Applications in Remote Sensing to Forest Ecology and Management’, One Earth. Elsevier Inc., 2(5), pp. 405–412. doi: 10.1016/j.oneear.2020.05.001.
McKenna P B, Lechner A M, Phinn S and Erskine P, 2020. ‘Remote Sensing of Mine Site Rehabilitation for Ecological Outcomes: A Global Systematic Review’, Remote Sensing, 12(21), pp. 1–34. doi: 10.3390/rs12213535.
Weiersbye, I M, Margalit N, Feingersh T, Revivo G, Stark R, Zur Y, Heller D, Braun O and Cukrowska EM 2006. ‘Use of airborne hyper-spectral remote sensing (HSRS) to focus remediation and monitor vegetation processes on gold mining landscapes in South Africa’, Mine Closure 2006, pp. 601–611.